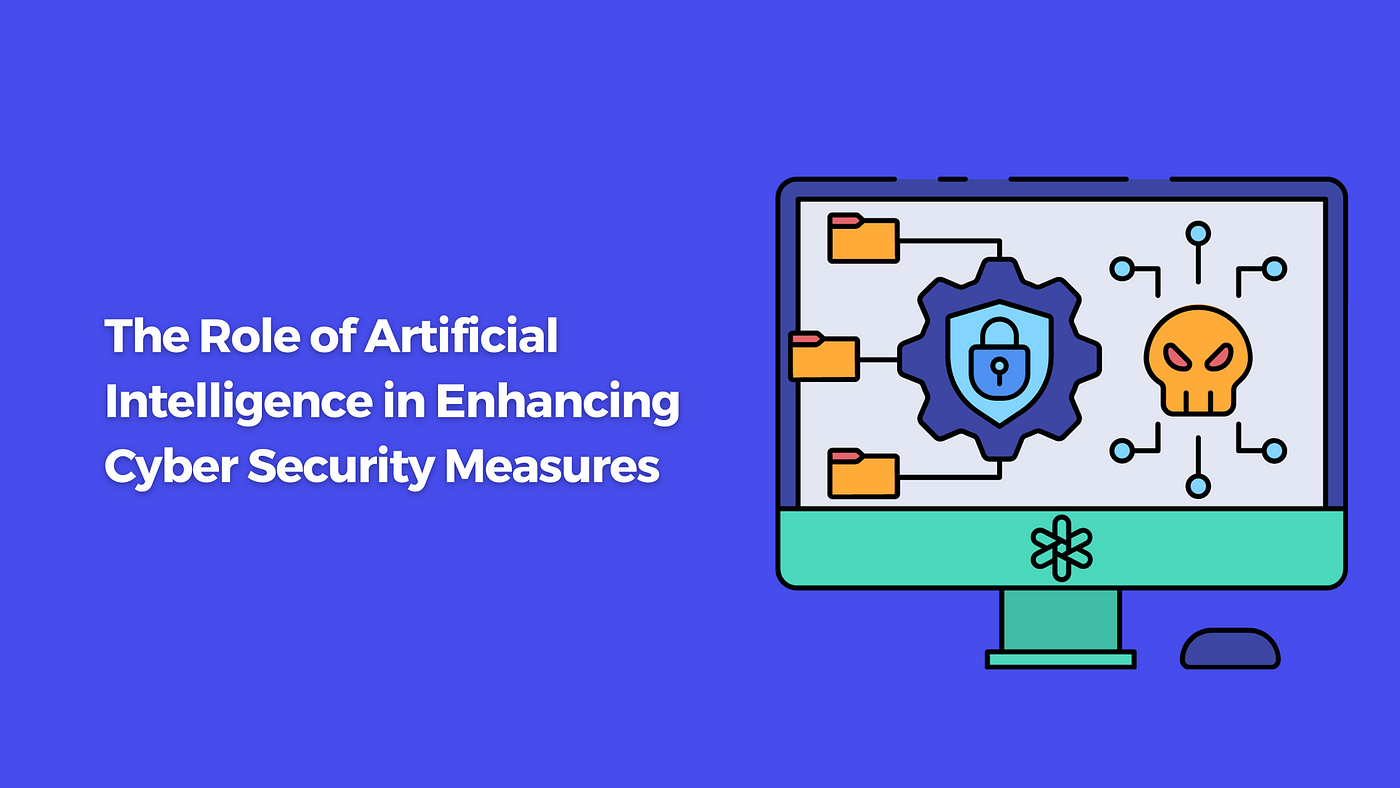
The Growing Need for AI in Cybersecurity
Cyber threats are becoming more sophisticated, with attackers using advanced techniques to bypass traditional security measures. Phishing attacks, malware, ransomware, and zero-day exploits are just a few examples of the ever-evolving threat landscape. Traditional cybersecurity tools, which rely on predefined rules and signatures, often fall short in detecting and mitigating these threats. As a result, there is an increasing need for more dynamic and adaptive solutions.
AI offers a promising solution by leveraging machine learning (ML), deep learning, and natural language processing (NLP) to detect and respond to threats in real time. AI-driven systems can analyze vast amounts of data from various sources, identify patterns, and predict potential threats before they materialize. This proactive approach is a game-changer in the fight against cybercrime.
AI-Powered Threat Detection
AI significantly enhances threat detection in cybersecurity. Traditional methods depend on known signatures and predefined rules for identifying threats. These methods often miss new or unknown threats. AI, however, analyzes large datasets to spot anomalies and patterns. This helps identify potential threats more effectively.
Behavioral Analysis:AI-driven systems can monitor user and network behavior to detect any deviations from the norm. For example, if an employee suddenly starts accessing sensitive files at odd hours or from an unusual location, AI can flag this behavior as suspicious. By continuously learning from user behavior, AI can detect and respond to threats in real time.
Anomaly Detection:AI can analyze network traffic to identify unusual patterns that may indicate a cyber attack. For instance, AI can detect an unusual spike in data transfer or a sudden increase in login attempts, which could be signs of a distributed denial-of-service (DDoS) attack or a brute force attack. By identifying these anomalies early, AI can help prevent attacks before they cause significant damage.
Predictive Analytics:AI can also be used for predictive analytics, where it analyzes historical data to predict future threats. By understanding the tactics, techniques, and procedures (TTPs) used by cybercriminals, AI can anticipate their next move and help organizations strengthen their defenses accordingly.
AI in Threat Response
Detecting threats is only half the battle; responding to them quickly and effectively is equally important. AI enhances threat response by automating processes, reducing response times, and minimizing the impact of cyber attacks.
Automated Incident Response:AI can automate many aspects of incident response, from identifying the threat to containing it and remediating the damage. For example, if AI detects a malware infection, it can automatically isolate the affected system, preventing the malware from spreading to other parts of the network. AI can also initiate automated remediation processes, such as rolling back changes made by the malware or restoring affected files from backups.
Real-Time Threat Mitigation:AI enables real-time threat mitigation by continuously monitoring network activity and responding to threats as they occur. For example, if AI detects a phishing attempt, it can automatically block the malicious email or website, preventing users from falling victim to the attack. This real-time response is crucial in minimizing the impact of cyber attacks.
Adaptive Security Measures:AI can adapt to new threats by continuously learning from its experiences. As cybercriminals evolve their tactics, AI systems can update their algorithms and models to stay ahead of the curve. This adaptive approach ensures that organizations are always protected against the latest threats.
AI in Vulnerability Management
Vulnerability management is a critical aspect of cybersecurity, and AI plays a vital role in identifying and addressing vulnerabilities before they can be exploited by attackers.
Vulnerability Scanning:AI can enhance vulnerability scanning by automating the process and making it more efficient. Traditional vulnerability scanning tools often produce a large number of false positives, overwhelming security teams with unnecessary alerts. AI can analyze scan results to prioritize vulnerabilities based on their severity and likelihood of exploitation, allowing security teams to focus on the most critical issues.
Predictive Vulnerability Management:AI can predict which vulnerabilities are most likely to be exploited by analyzing historical data and trends. For example, AI can identify patterns in how cybercriminals exploit certain types of vulnerabilities and use this information to predict which vulnerabilities are most at risk. This predictive approach helps organizations prioritize their patching efforts and reduce their attack surface.
Patch Management:AI can also streamline the patch management process by automating the deployment of patches and updates. AI can identify which systems are vulnerable, prioritize patches based on the severity of the vulnerabilities, and deploy the patches in a timely manner. This automation reduces the risk of human error and ensures that vulnerabilities are addressed promptly.
AI in Fraud Detection
AI significantly impacts fraud detection, especially in financial institutions, e-commerce platforms, and organizations handling sensitive data. Fraudsters constantly target these sectors. AI-driven systems detect and prevent fraud by analyzing transaction patterns, user behavior, and other indicators. This highlights how AI is enhancing cybersecurity measures.
Transaction Monitoring:AI monitors transactions in real-time to detect suspicious activity. For example, when a credit card is suddenly used in multiple locations, AI flags it as potential fraud, alerts the cardholder, or blocks the transaction. This real-time monitoring highlights how AI is enhancing cybersecurity measures by preventing fraud before it causes significant financial losses.
User Authentication:AI can enhance user authentication by analyzing behavioral biometrics, such as typing patterns, mouse movements, and even the way a user holds their device. By comparing these patterns to the user’s known behavior, AI can determine whether the person attempting to access the system is legitimate or a fraudster. This additional layer of security makes it more difficult for attackers to bypass authentication mechanisms.
Anomaly Detection in Financial Transactions:AI can identify anomalies in financial transactions that may indicate fraudulent activity. For example, AI can detect unusual spending patterns, such as a sudden increase in purchases from a specific location or a change in the type of goods being purchased. By flagging these anomalies, AI can help organizations prevent fraud and protect their customers’ financial information.
Challenges and Considerations
AI offers significant benefits in enhancing cybersecurity measures but also presents challenges. A primary concern is cybercriminals potentially using AI to launch more sophisticated attacks. For instance, AI-powered malware can adapt to security measures in real-time, making it difficult to detect and mitigate.Ethical considerations are another challenge. AI systems rely on the data they are trained on. Biased or incomplete data can lead to inaccurate predictions and decisions. Organizations must train AI systems on diverse, representative data to avoid potential biases.
Trust is also a crucial issue. As AI integrates into cybersecurity, organizations must ensure transparency and explainability in their AI systems. Users need to understand AI’s decision-making process and trust that those decisions are accurate and fair.
Finally, AI can detect anomalies in financial transactions, identifying unusual spending patterns or changes in purchase types. Flagging these anomalies helps prevent fraud and protect customers’ financial information.
Conclusion
AI is revolutionizing cybersecurity by enhancing threat detection, response, and prevention. It analyzes vast amounts of data, detects anomalies, and adapts to new threats, making it an invaluable tool in the fight against cybercrime. However, organizations must consider the challenges and ethical implications of using AI in cybersecurity. Addressing these challenges and leveraging AI’s full potential allows organizations to significantly improve their cybersecurity measures and protect their digital assets in an increasingly complex threat landscape.
Author:Golvez Technology