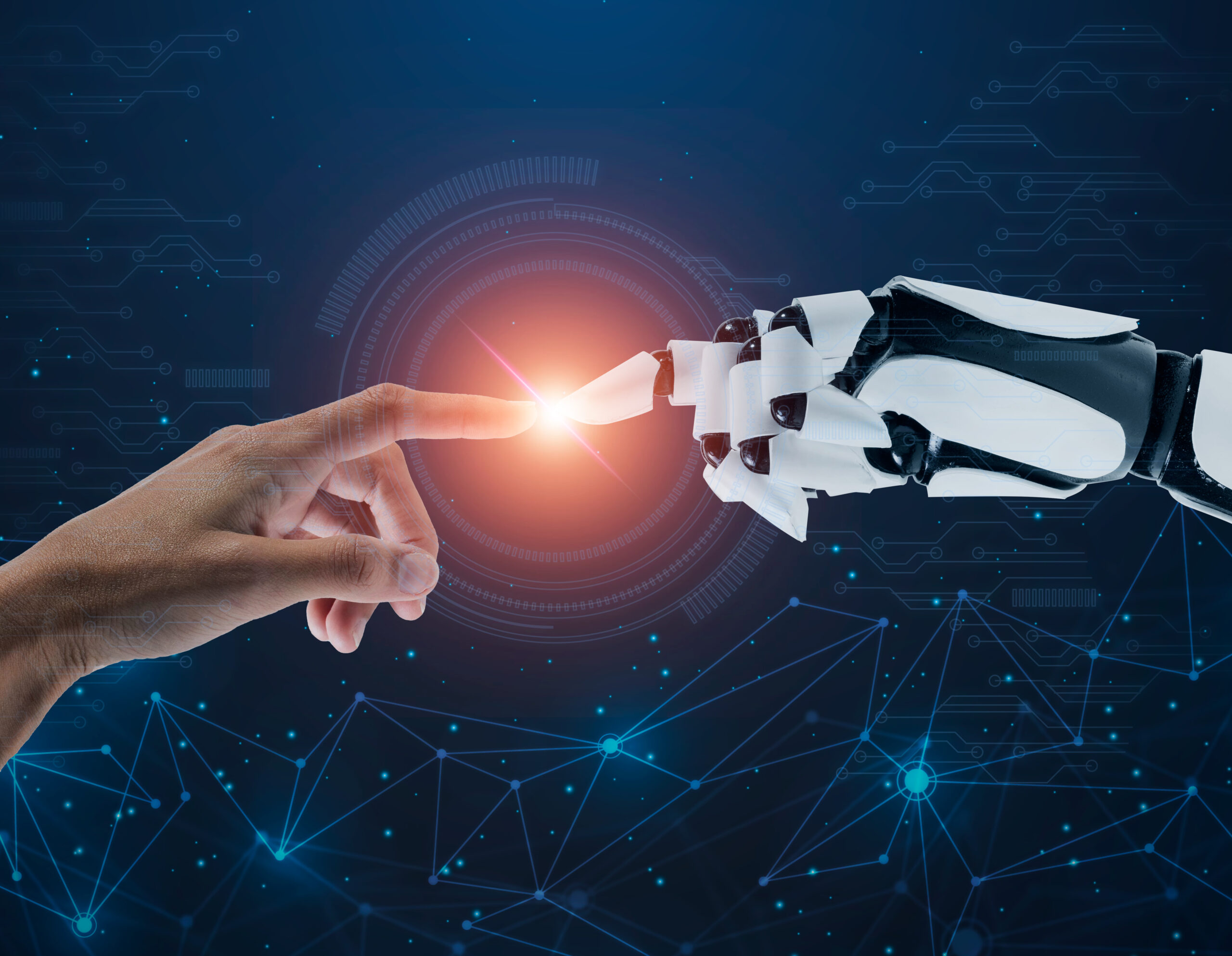
Deep Learning
Deep learning represents a subset of machine learning and artificial intelligence (AI) that seeks to replicate human-like learning and understanding. These advanced models can master complex tasks, such as classifying data and identifying patterns in images, text, audio, and other forms of data. Additionally, they can automate tasks that typically require human intelligence, like providing descriptions for images or transcribing audio files.
In the context of data science, deep learning plays a pivotal role alongside statistics and predictive modeling. Moreover, this technology greatly benefits data scientists in gathering, analyzing, and deciphering vast volumes of data. Consequently, deep learning accelerates and streamlines this process, offering a faster and more efficient means of data exploration and insight generation.
Furthermore, it has become an essential tool in the data scientist’s toolkit, particularly in exploring the applications of deep learning. This technology pushes the boundaries of what is achievable in data analysis and predictive modeling, enabling more profound insights and innovative solutions..
Why is deep learning important?
Deep learning demands two key resources: abundant labeled data and substantial computing power. With these resources, deep learning becomes highly effective. It can be applied in various areas like digital assistants, fraud detection, and facial recognition. What’s remarkable about deep learning is its exceptional accuracy, which is crucial in safety-critical applications. For example, it plays a vital role in autonomous vehicles and medical devices, where precision is essential.
In simpler terms, with the right data and computing capabilities, deep learning excels in tasks requiring high precision. This makes it indispensable in many critical areas.
Deep Learning Technology Applications
Customer Service: Many organisations are embracing machine learning to enhance their customer service. A common example is chatbots, which you’ll find on customer service websites and apps. These bots have evolved, becoming smarter and capable of handling complex queries. They learn to provide answers, even when questions are a bit unclear. Additionally, virtual assistants like Siri, Alexa, and Google Assistant are prime examples of how deep learning is applied to create intelligent and helpful tools. These innovations make interactions with technology more intuitive and user-friendly.
Health Care Industry: Deep learning technology has made a significant impact on the healthcare sector. Many healthcare organisations have transitioned to digital record-keeping and imaging to enhance efficiency and reduce manual errors. Moreover, the integration of image recognition has sped up the analysis and evaluation of a large volume of medical images, significantly reducing the time required for diagnosis and treatment planning. This transformation is improving patient care and outcomes in the healthcare industry.
Finance Industry: Last but certainly not least, the application of predictive analytics within financial institutions has brought forth a range of invaluable advantages. These benefits encompass the detection of fraudulent activities, the evaluation of business risks to inform loan approval decisions, and the implementation of algorithmic trading strategies in the stock market. Through predictive analytics, financial institutions have gained the ability to make more informed, data-driven decisions, ultimately leading to improved risk management, enhanced security, and optimised investment strategies.
Author : Golvez Technology