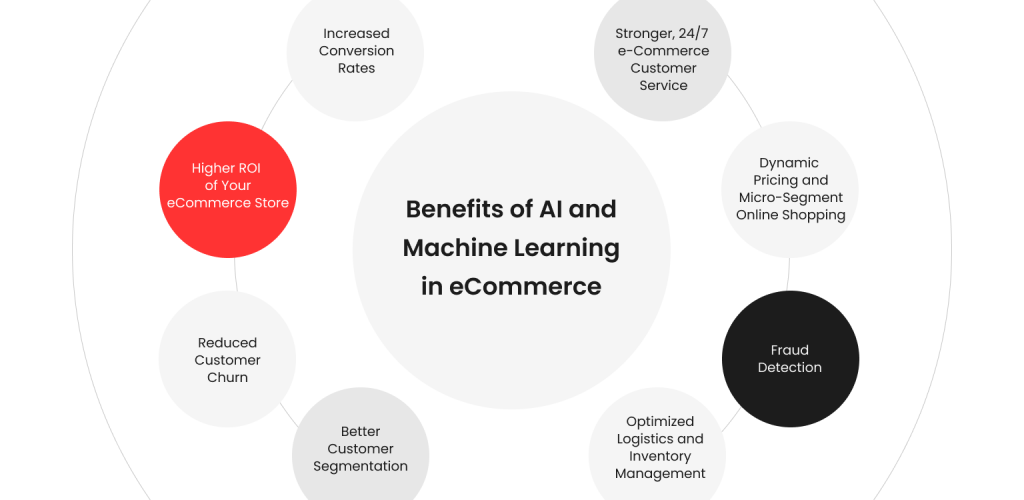
1. Personalization at Scale
Machine learning allows eCommerce platforms to deliver personalized recommendations. By analyzing browsing history, purchasing patterns, and customer preferences, machine learning algorithms can suggest products that suit individual tastes, making customers more likely to engage and make purchases.
Benefit:
Personalized product recommendations lead to increased sales and improved customer loyalty.
Use Case:
Amazon utilizes machine learning to tailor its product recommendations to millions of users, generating a significant portion of its revenue through personalized suggestions.
Best Practice:
Integrate machine learning-driven recommendation engines to track user behavior in real time and update suggestions dynamically. This personalization boosts engagement and encourages repeat purchases.
2. Improved Customer Service with Chatbots and Virtual Assistants
Chatbots powered by machine learning enhance customer support by providing instant answers to customer queries. These intelligent bots can understand and respond to common questions, reducing the need for human intervention.
Benefit:
Chatbots provide 24/7 support, reduce response times, and lower customer service costs.
Use Case:
Sephora’s chatbot helps customers find products, offers makeup tutorials, and guides them through purchase processes. It engages users and improves the overall shopping experience.
Best Practice:
Ensure chatbots are regularly updated with new customer interactions and data to continuously improve response accuracy and customer satisfaction.
3. Dynamic Pricing for Competitive Advantage
Dynamic pricing powered by machine learning helps eCommerce businesses stay competitive by adjusting product prices based on real-time factors like demand, competitor pricing, and customer behavior.
Benefit:
Dynamic pricing maximizes revenue by ensuring the right price at the right time.
Use Case:
Uber uses machine learning algorithms to implement surge pricing, increasing prices based on demand and maximizing profits during peak times.
Best Practice:
Implement dynamic pricing strategies across product categories, factoring in real-time data from competitors and customer activity.
4. Fraud Detection and Prevention
Machine learning enables faster and more accurate fraud detection by identifying unusual patterns and anomalies in transactions. It can distinguish between legitimate and fraudulent activities, protecting businesses from financial losses.
Benefit:
ML-based fraud detection minimizes chargebacks, reduces fraudulent activity, and enhances trust.
Use Case:
PayPal uses machine learning to monitor millions of transactions daily and detect suspicious patterns in real-time, resulting in reduced fraud rates.
Best Practice:
Incorporate machine learning fraud detection systems that continuously learn from new data to detect evolving threats effectively.
5. Inventory and Supply Chain Optimization
Machine learning algorithms optimize inventory management by predicting demand, minimizing overstock and understock situations, and improving supply chain efficiency.
Benefit:
Better inventory management reduces costs, minimizes waste, and improves operational efficiency.
Use Case:
Zara uses machine learning to predict fashion trends and adjust its inventory levels accordingly, reducing excess stock and boosting profitability.
Best Practice:
Use predictive analytics to monitor trends and sales patterns, ensuring that stock levels align with demand, leading to fewer out-of-stock issues and wasted resources.
6. Enhanced Search and Navigation
Machine learning improves search algorithms, making it easier for customers to find products quickly and efficiently. By understanding natural language queries and learning from search behavior, it enhances user experience.
Benefit:
Improved search accuracy leads to higher conversion rates and increased user satisfaction.
Use Case:
eBay uses machine learning to optimize its search engine, helping users find relevant products even with complex or vague search queries.
Best Practice:
Leverage natural language processing (NLP) to enhance search functionality. This ensures that product results are relevant, regardless of how customers phrase their queries.
7. Predictive Analytics for Marketing Campaigns
By analyzing customer behavior and historical data, machine learning enables businesses to predict which marketing campaigns will be most effective. It helps in targeting the right audience, delivering personalized messages, and improving conversion rates.
Benefit:
Predictive analytics enables smarter marketing decisions, leading to higher returns on investment (ROI).
Use Case:
Netflix uses machine learning to predict what types of content users are likely to enjoy based on their viewing habits, helping them market shows more effectively.
Best Practice:
Use machine learning to continuously optimize marketing campaigns, focusing on personalized messaging based on user preferences and historical data.
8. Optimizing Logistics and Delivery
Machine learning can streamline logistics and delivery by predicting delivery times, optimizing routes, and improving last-mile delivery efficiency. This ensures that products reach customers faster, improving satisfaction.
Benefit:
Optimized logistics reduce delivery times, lower transportation costs, and enhance customer satisfaction.
Use Case:
FedEx uses machine learning to analyze delivery routes, weather conditions, and traffic patterns to optimize shipping and reduce delays.
Best Practice:
Implement machine learning algorithms to optimize delivery routes and schedules, ensuring faster, more cost-effective shipping.
9. Customer Sentiment Analysis
Machine learning enables businesses to analyze customer reviews and feedback, gaining insights into customer sentiment. This helps in identifying areas for improvement, understanding customer preferences, and enhancing overall satisfaction.
Benefit:
Sentiment analysis improves customer experience and helps businesses address negative feedback effectively.
Use Case:
Nike uses machine learning to analyze customer reviews on its website, gaining insights into product preferences and potential improvements.
Best Practice:
Use sentiment analysis to continuously monitor customer feedback and address concerns, ensuring that product quality and customer service improve.
10. Cross-Selling and Upselling Opportunities
Machine learning identifies cross-selling and upselling opportunities by analyzing customer purchasing patterns and predicting what additional products they might want. This increases average order value (AOV) and drives additional revenue.
Benefit:
Cross-selling and upselling boost sales and improve the overall shopping experience.
Use Case:
Amazon frequently suggests complementary products during the checkout process, using machine learning to increase AOV.
Best Practice:
Incorporate machine learning algorithms that recommend relevant products during the purchase process, increasing the chances of upselling and cross-selling.
Best Practices for Implementing Machine Learning in eCommerce
To effectively harness the power of machine learning in eCommerce, businesses should follow best practices that ensure successful integration and long-term benefits.
1. Start Small, Scale Gradually2. Leverage Quality Data
The success of machine learning models relies heavily on data quality. Ensure that the data used for training algorithms is accurate, diverse, and up-to-date.
3. Collaborate with Data Scientists
Work closely with data scientists to develop, fine-tune, and optimize machine learning models. Their expertise is essential in understanding complex algorithms and ensuring they align with business goals.
4. Continuously Train Models
Machine learning models need to evolve with changing customer behaviors and market trends. Regularly update and retrain models to improve accuracy and relevance.
5. Integrate Across Platforms
For maximum impact, integrate machine learning solutions across multiple platforms, including websites, mobile apps, and email marketing campaigns. Consistency across channels enhances customer experience and drives engagement.
6. Prioritize Customer Privacy
While machine learning requires vast amounts of data, businesses must ensure that customer privacy remains a priority. Implement strict data security measures and be transparent about how customer data is used.
7. Monitor Performance
Continuously track the performance of machine learning models to ensure they meet key performance indicators (KPIs). Regular monitoring helps identify areas for improvement and keeps models aligned with business goals.
Conclusion
Machine learning is reshaping the eCommerce landscape, offering numerous benefits that improve customer experiences, optimize operations, and increase revenue. From personalized recommendations to fraud detection and inventory optimization, machine learning enhances every aspect of the online shopping journey. By following best practices such as leveraging quality data, collaborating with data scientists, and continuously training models, businesses can harness the full potential of machine learning in eCommerce. The future of eCommerce lies in data-driven, intelligent solutions, and companies that embrace this technology will continue to thrive in an increasingly competitive market.
Embracing machine learning is not just a trend but a necessity for businesses looking to stay ahead in the digital era. By strategically integrating machine learning technologies, eCommerce platforms can deliver enhanced value to customers and drive sustainable growth.